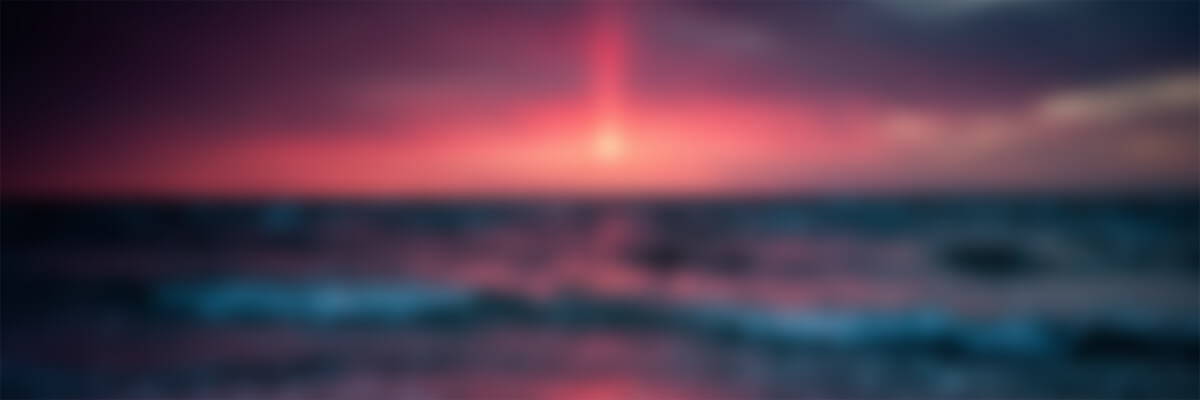
通义千问artificial intelligence
A2: Although it is generally believed that the explanatory nature of the model helps to improve user trust, the experimental results show that this enhancement is not significant and not as effective as feedback. In specific cases, such as areas of low expertise, some form of interpretation may result in only a modest increase in appropriate trust.
- MIT Licensed | Copyright © 2024-present Zhirong Xue's knowledge base20202021A3: The study found that the feedback of the results can improve the accuracy of the user's predictions (reducing the absolute error), thereby improving the performance of working with AI. However, interpretability does not have as much impact on user task performance as it does on trust. This may mean that we should pay more attention to how to effectively use feedback mechanisms to improve the usefulness and effectiveness of AI-assisted decision-making.4.1KThe Interpretability of Artificial Intelligence and the Impact of Outcome Feedback on Trust: A Comparative StudyAItooltoggle sidebarThe Interpretability of Artificial Intelligence and the Impact of Outcome Feedback on Trust: A Comparative Study Alt_BlogApplewatchhttps://dl.acm.org/doi/10.1145/3613904.3642780CentOS7CorePressMiX Copilothttps://dl.acm.org/doi/10.1145/3613904.3642780CtrlMiX CopilotBased on large language model generation, there may be a risk of errors.ios14ios14.2iPhone 11iPhone 12iphone 12 MaxiPhone 12 proiphone 12miniiPhone SEiphone12 proSearchmacosmemcachedofficeonedriveOriginal address:AItoggle sidebarXRXRSurfaceFleetHCIPersonal insightsQ1: How does feedback affect users' trust in AI?WWDCwin10WindowswordpWordPressWP-China-YesTranslationXue Zhirong, Designer, Interaction Design, Human-Computer Interaction, Artificial Intelligence, Official Website, Blog, Creator, Author, Engineer, Paper, Product Design, Research, AI, HCI, Design, Learning, Knowledge Base, xuezhirong, UX, Design, Research, AI, HCI, Designer, Engineer, Author, Blog, Papers, Product Design, Study, Learning, User ExperienceDraw inferences中国抗疫速度中国移动中国高铁thesis云卷云舒云服务器云虚拟主机User experienceInteractiveA1: According to research, feedback (e.g. result output) is a key factor influencing user trust. It is the most significant and reliable way to increase user trust in AI behavior.summary京雄城际铁路solutionThe content is made up of:免费主机免费服务器免费空间Q3: How does result feedback and model interpretability affect user task performance?安装win10The Interpretability of Artificial Intelligence and the Impact of Outcome Feedback on Trust: A Comparative Study宝塔面板实时疫情The Interpretability of Artificial Intelligence and the Impact of Outcome Feedback on Trust: A Comparative Study Xue Zhirong is a designer, engineer, and author of several books; Founder of the Design Open Source Community, Co-founder of MiX Copilot; Committed to making the world a better place with design and technology. This knowledge base will update AI, HCI and other content, including news, papers, presentations, sharing, etc.Xue Zhirong, Designer, Interaction Design, Human-Computer Interaction, Artificial Intelligence, Official Website, Blog, Creator, Author, Engineer, Paper, Product Design, Research, AI, HCI, Design, Learning, Knowledge Base, xuezhirong, UX, Design, Research, AI, HCI, Designer, Engineer, Author, Blog, Papers, Product Design, Study, Learning, User Experienceartificial intelligenceDissertation SummaryA1: According to research, feedback (e.g. result output) is a key factor influencing user trust. It is the most significant and reliable way to increase user trust in AI behavior.solutionThe Interpretability of Artificial Intelligence and the Impact of Outcome Feedback on Trust: A Comparative Study | Xue Zhirong's knowledge baseProblem findingThe researchers conducted two sets of experiments ("Predict the speed-dating outcomes and get up to $6 (takes less than 20 min)" and a similar Prolific experiment) in which participants interacted with the AI system in a task of predicting the outcome of a dating to explore the impact of model explainability and feedback on user trust in AI and prediction accuracy. The results show that although explainability (e.g., global and local interpretation) does not significantly improve trust, feedback can most consistently and significantly improve behavioral trust. However, increased trust does not necessarily lead to the same level of performance gains, i.e., there is a "trust-performance paradox". Exploratory analysis reveals the mechanisms behind this phenomenon.The researchers conducted two sets of experiments ("Predict the speed-dating outcomes and get up to $6 (takes less than 20 min)" and a similar Prolific experiment) in which participants interacted with the AI system in a task of predicting the outcome of a dating to explore the impact of model explainability and feedback on user trust in AI and prediction accuracy. The results show that although explainability (e.g., global and local interpretation) does not significantly improve trust, feedback can most consistently and significantly improve behavioral trust. However, increased trust does not necessarily lead to the same level of performance gains, i.e., there is a "trust-performance paradox". Exploratory analysis reveals the mechanisms behind this phenomenon.The researchers found that although it is generally believed that the interpretability of the model can help improve the user's trust in the AI system, in the actual experiment, the global and local interpretability does not lead to a stable and significant trust improvement. Conversely, feedback (i.e., the output of the results) has a more significant effect on increasing user trust in the AI. However, this increased trust does not directly translate into an equivalent improvement in performance.Q1: How does feedback affect users' trust in AI?To assess trust more accurately, the researchers used behavioral trust (WoA), a measure that takes into account the difference between the user's predictions and the AI's recommendations, and is independent of the model's accuracy. By comparing WoA under different conditions, researchers can analyze the relationship between trust and performance.
MIT Licensed | Copyright © 2024-present Zhirong Xue's knowledge base
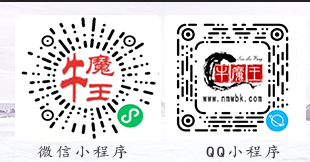
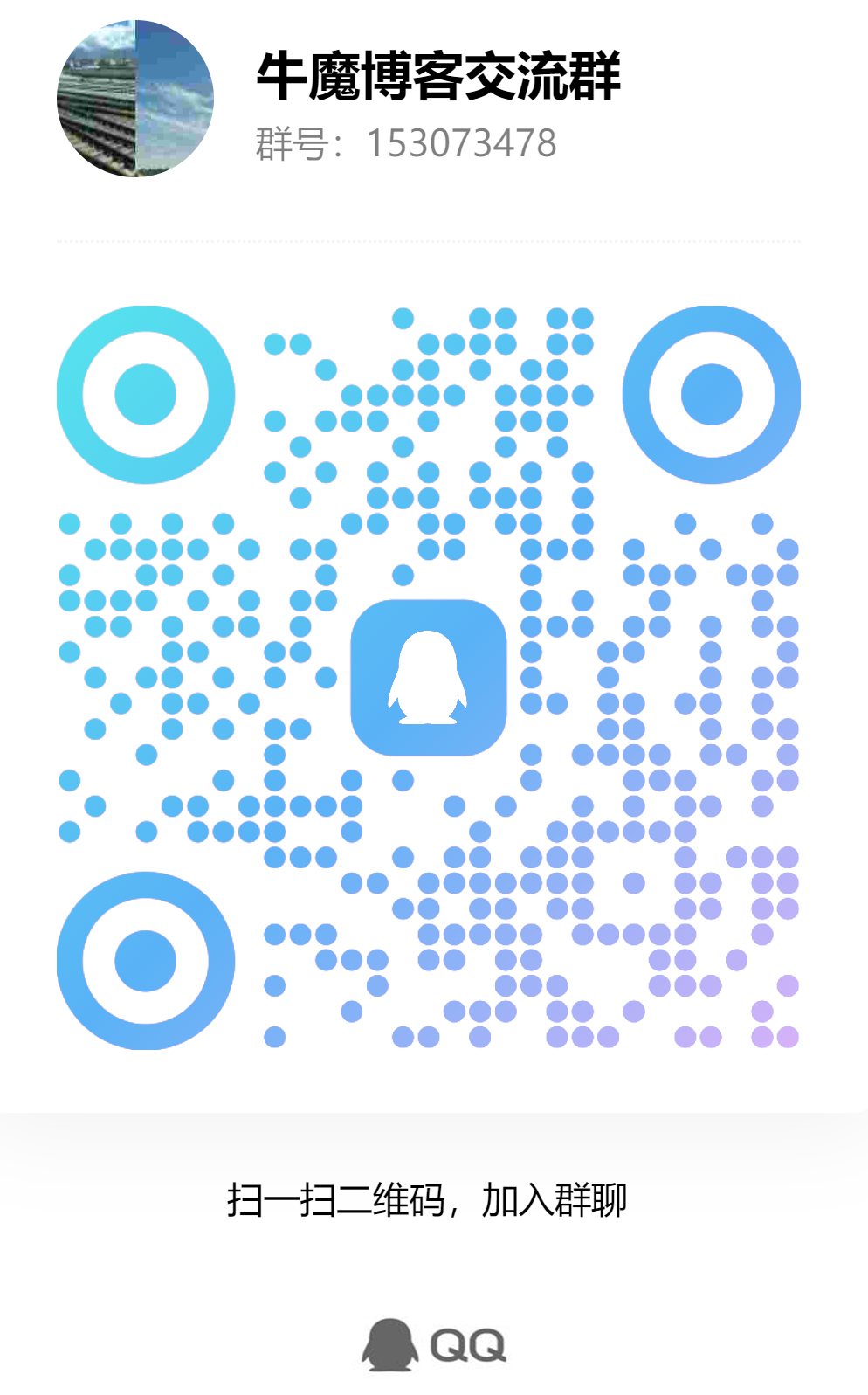
The content is made up of:
五一劳动节
24 天
2025-05-01
speech
21.62%
还剩 19 小时
Robots and digital humans
14.29%
还剩 6 天
Q2: Does explainability necessarily enhance users' trust in AI?
20.00%
还剩 24 天
To assess trust more accurately, the researchers used behavioral trust (WoA), a measure that takes into account the difference between the user's predictions and the AI's recommendations, and is independent of the model's accuracy. By comparing WoA under different conditions, researchers can analyze the relationship between trust and performance.
26.30%
还剩 269 天
摸鱼日报
Robots and digital humans
牛魔博客WordPressartificial intelligencewin10The content is made up of:About me子比主题outcome2021阿里云盘InterviewQ2: Does explainability necessarily enhance users' trust in AI?Original address:Xue Zhirong is a designer, engineer, and author of several books; Founder of the Design Open Source Community, Co-founder of MiX Copilot; Committed to making the world a better place with design and technology. This knowledge base will update AI, HCI and other content, including news, papers, presentations, sharing, etc.summary
The researchers found that although it is generally believed that the interpretability of the model can help improve the user's trust in the AI system, in the actual experiment, the global and local interpretability does not lead to a stable and significant trust improvement. Conversely, feedback (i.e., the output of the results) has a more significant effect on increasing user trust in the AI. However, this increased trust does not directly translate into an equivalent improvement in performance.
网站信息统计
- 文章总数:1185 篇
- 评论数目:77 条
- 标签总数:1662 个
- 浏览次数:1814036 次
- 友链总数:43 个
- 用户总数:5901 个
- 运行天数:1995 天
- 建站时间:2019-10-21
- 最后更新:2025-04-07
- 数据查询:23 次
- 生成耗时:0.82511秒